A new take on melanoma diagnosis
Source: Medical Physics Web, May 2015
Colour is an important characteristic for diagnosing skin lesions such as malignant melanoma. In dermatological images, however, the colour is affected not only by melanin and haemoglobin under the skin surface, but also by the presence of external illumination. If pigmentation data are affected by illumination artefacts, conventional colourimetry may not accurately describe the skin histology and disease may be incorrectly diagnosed.
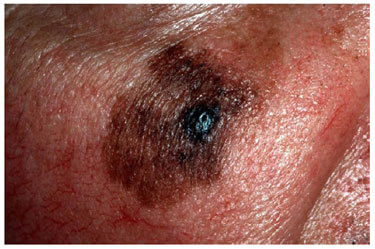
Sourcee: Medical PhysicsWeb
To address this issue, Zhao Liu and Josiane Zerubia from INRIA in France have developed a new illumination modelling and chromophore identification method that corrects for lighting variations in skin colour images. The method also determines the concentrations of melanin and haemoglobin in the skin (Phys. Med. Biol. 60 3415).
“Skin images corrected by the proposed method greatly improve the quality of clinical images captured under non-calibrated light settings," explained Liu (currently based at Manchester University). “This can help dermatologists achieve more trustworthy diagnostic results."
Three-step model
In contrast to existing illumination correction approaches, which only address diffuse skin reflection, Liu and Zerubia propose a method that considers both diffuse and specular reflections in a multi-layered skin model.
“This allows our model to address both highlight and strong shading effects," said Liu.
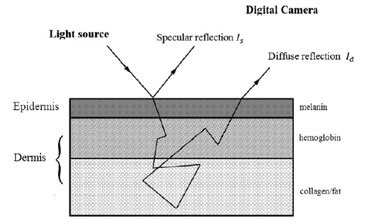
Source: MedicalPhysicsWeb
The model works by first separating specular from diffuse reflection. To do this, the researchers used a thresholding method to identify pixels likely to contain a specular component, and then employed a B-spline interpolation (rather than direct subtraction, which may yield some fake intensity pixels) to extract the diffuse reflection from the original image.
The diffuse image is then split into a base layer and a detail layer. The base layer, which contains low-frequency illumination and shading information, is approximated by polynomial curve fitting. The detail layer, which contains the high-frequency chromophore reflectance, is then calculated by subtracting the base layer from the diffuse spectral band in a log form.
Finally, the melanin and haemoglobin density are determined based on knowledge of their chromophore absorption spectra. Due to the increased attenuation of haemoglobin, image intensity in the red channel (around 650 nm) for a conventional RGB camera is primarily attributed to melanin, which effectively absorbs light from 400 to 1000 nm. Image intensities in the green (550 nm) and blue (450 nm) channels are joint effects of melanin and haemoglobin (oxyhaemoglobin and deoxyhaemoglobin absorb light at around 450 nm and 570 nm).
By analysing data from the different spectral channels, the researchers calculated a melanin index (MI) and a haemoglobin index (HI) for use in lesion diagnosis. These indices, which relate directly to pathological tissue conditions, tend to be less influenced by external lighting.
Lesion segmentation
To evaluate their proposed algorithm, Liu and Zerubia examined 258 conventional RGB skin lesion images, including 76 malignant melanoma and 182 benign nevi, from public databases. They first performed a lesion segmentation experiment, comparing images after modelling with the new algorithm with results from two state-of-the-art illumination correction methods (Comput. Med. Imag. Graph. 35 481 and IEEE Trans. Biomed. Eng. 60 1873) developed for skin image analysis.
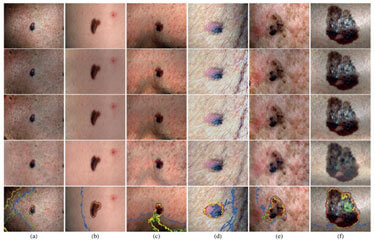
SDource:MedicalPhysicsWeb
Visual examination of example images after illumination correction revealed that the two alternative schemes caused colour changes in shaded areas, whilst the new method maintained a consistent chromaticity of healthy skin. Furthermore, for skin images with complex surface shapes and oversaturated illumination variations, the proposed method was the only one that could successfully remove the shading effects.
After illumination modelling, the skin images were automatically segmented into areas of lesion and non-lesion, and the results compared with manual segmentation by an experienced dermatologist. The researchers evaluated the segmentation accuracy using the Tanimoto coefficient (TC), which ranges from 100% if the two segmentations match exactly, to 0% when there is no overlap.
For an uncorrected skin lesion image, the average TC was 67.54%. The state-of-the-art illumination modelling algorithms increased this to 80.63% and 82.78%, while the newly proposed method exhibited superior performance with an average TC of 88.96%.
Melanoma diagnosis
To demonstrate the efficiency of the chromophore indices for skin disease analysis, Liu and Zerubia compared computer-aided melanoma classification using diagnostic features extracted from MI and HI with features extracted from red, green and blue channels for RGB colourimetry (before and after illumination correction).
For classification in RGB colourimetry, the new illumination correction method exhibited a sensitivity in differentiating malignant melanoma from benign nevi of 73.68%, up from 65.78% for uncorrected images. The two state-of-the-art methods achieved 69.74% and 71.05% sensitivity. The new method achieved 89.56% specificity, compared with 68.67% (uncorrected) and 76.92% and 80.77% (state-of-the-art methods).
Applying diagnostic descriptors derived from MI and HI improved the sensitivity of automatic melanoma diagnosis by a further 12%, with 5% sacrifice in specificity.
Liu and Zerubia concluded that, as the main objective for melanoma diagnosis is to maximize correct recognition of malignant lesions, the best classification is achieved using the new illumination modelling method with the chromophore descriptors. This combination provided 85.52% sensitivity, 84.07% specificity and 84.50% overall diagnostic accuracy.
Next, they plan to optimize the algorithm to run in real time, offering the potential to use the proposed method in clinical practice. They are also looking at the possibility of applying the approach to diagnosis of other skin diseases. “We have done an initial study on automatic acne detection using haemoglobin descriptors, in collaboration with Centre Hospitalier Universitaire de Nice," said Liu.